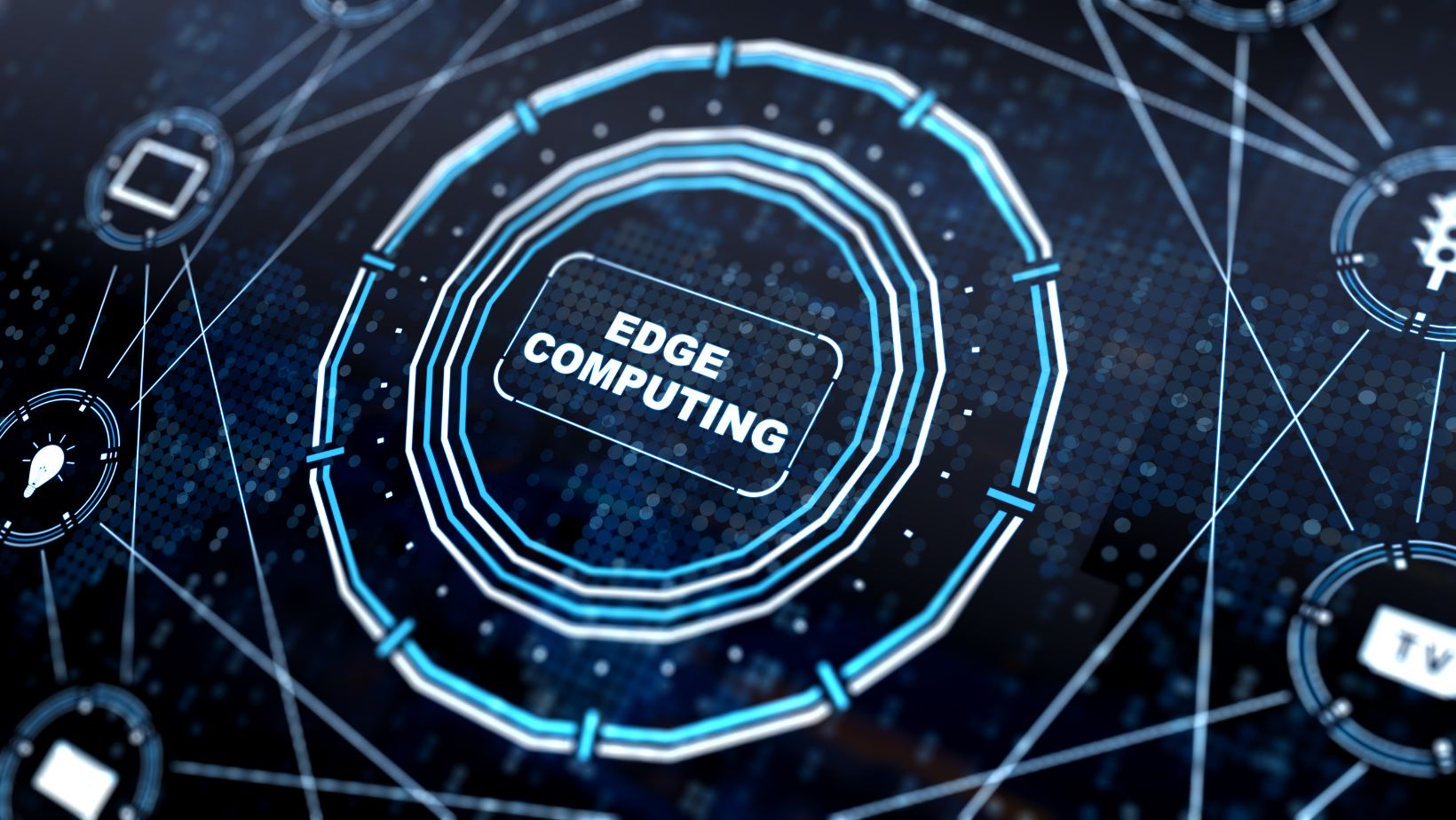
The continuous evolution of the technology landscape has taken a new turn following the launch of Edge AI. With different industries embracing the complex nature of data today the importance of Edge AI is now more evident as it helps in reshaping business analysis, decision making and automations.
This shift also brings the capabilities of AI closer to data generation sources and this further allows for real time insights and processing. Memory ICs play a key role in this transformation, providing the necessary storage and fast access to data which is vital for efficient Edge AI operations. In this article we will take you through how the integration of AI with Edge computing revolutionizes local data processing and modern industries.
A Brief Overview of Edge AI
Edge AI is an innovative technology that utilizes AI algorithms on edge devices like IoT enabled gadgets and industrial computers. This integration allows for the performance of real time data analysis and processing especially at the point where the data is generated.
Unlike with conventional AI models that function by sending data to a central cloud or server for analysis, the need for data to travel long distances is not required with Edge AI and this allows for reduced bandwidth usage and faster response time. One of the importance of Edge AI is that it can be utilized within the transport sector for the analysis of traffic patterns and adjustments of traffic lights. This helps in improving the flow of traffic and reducing congestions.
Another function can be seen in surveillance where there’s need for real time video feeds analysis for the detection of suspicious activities. The manufacturing sector also benefits from Edge AI and this is through its use in process optimization, quality control and predictive maintenance.
There are so many other benefits of Edge AI and this includes data processing for enhanced data security and quicker response to help minimize cyber attacks. Also Edge AI can be used with connectivity intermittently which makes it an ideal option for remote environments with poor internet access.
Types of Edge Computing
Edge AI can be classified in different ways according to data location and technology devices.
Here’s an overview of the types of edge computing:
Edge Computing Types by Technology Devices
Here are the types of edge computing by technology devices:
- Sensor Edge: The focus of this edge computing is on processing data at sensor level. This is key for IoT environments where there’s need for the generation of large data and analysis of this data in real time.
- Compute Edge: This edge computing utilizes local data or server centers to process data close to the source of the data. This is key for cases requiring more storage capacity and computational power.
- Cloud Edge: In this case data process is in a cloud network within close proximity to the source of data. This helps to create a balance between cloud resources and local processing.
- Device Edge: Device edge focuses on data analysis and process and the source of data. This is mostly within individual sensors or devices and it’s key for cases that requires swift responses.
Edge Computing Types by Data Location
Here are the types of edge computing by data location:
- Industrial Edge: This type is mostly applied in industrial environments because it’s vital for quality control, predictive maintenance and automation especially in cases that require real time data processing.
- Enterprise Edge: This is mostly within the local network of organizations thereby enhancing data processing near the data source. This is vital for reducing data transmission to external servers and increasing security.
- Provider Edge: In this case data processing is close to the service provider’s network and this approach is important for enhancing content delivery and other services.
Benefits of Integrating AI with Edge
With everything above here are the key benefits of integrating AI with Edge:
- Scalability: Edge computing facilitates easy resource scaling to match dynamic demands.
- Higher Speeds: Processing data locally helps in reducing latency and this makes Edge AI important in cases requiring fast response.
- Reduced Bandwidth Usage: Edge Ai reduces the number of data to be transferred to servers and this helps in reducing costs and bandwidth usage.
- Improved Data Security: Another benefit of Edge AI is that it minimizes the need for data transfer to external servers which further improves data compliance and security.
- Improved Reliability: Edge AI helps in reducing single failure points and this further enhance reliability of systems.
- Real-Time Analytics and Decision Making: Edge AI facilitates fast data analysis and processing at the data source and this enhances decision making.
Exploring the Implementation Edge AI
Edge devices are designed to capture data in real time and this is usually inconsistent. This is a challenge when it comes to ensuring reliability, integrity and data quality. With this there’s need for the development of technologies and strategies that can handle the unique features of data generated using edge.
Scalability is also a challenge which is why it’s important for organizations to design systems that can handle the demand for storage capacity, network bandwidth and computational resources. It’s worth noting that this might involve the utilization of edge data centers, implementing technologies like orchestration and containerization for more flexible scaling and the adoption of computing architectures. To effectively manage these challenges and enhance scalability, Transform your data strategy with robust solutions that streamline and optimize data flow.
Another challenge to note with the implementation of edge AI is security as the deployment of edge devices is mostly in physically accessible locations which are susceptible to various security threats. It’s important for organizations to implement various security measures like access controls, authentication, intrusion detection systems and encryption.
Conclusion
With technological advances it’s clear that edge computing will become more robust allowing for easy integration with machine learning and artificial intelligence to enhance the delivery of higher capabilities and efficiencies. Today 5G is becoming widely available globally and this is sure to boost the full scale adoption of edge computing because of more reliable and faster connectivity.
The bottom line is that edge computing is a key advancement in data processing because it helps in optimizing bandwidth, support scalability, deliver real time insights and enhance security. This further makes it a game changer for enterprises globally.
+